Artificial Intelligence – The rise of the machines
There is an innate fear of autonomous systems able to think for themselves, a fear that Hollywood has tapped into with great effect over the years, with films such as 2001: A Space Odyssey, Terminator and I, Robot. Whilst our worst fears are almost certainly unfounded, as Artificial Intelligence (AI) continues to develop, both in its capability and application, just how worried should we be and what can we do about it?
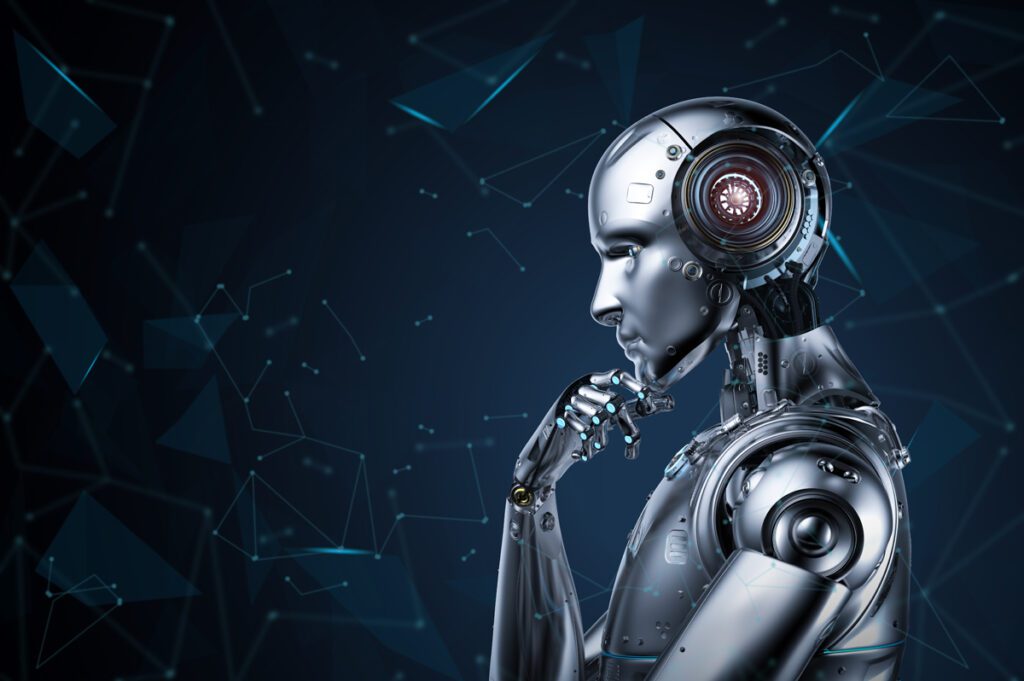
In its widest sense, the term AI encompasses computer systems able to perform tasks normally associated with human intelligence, such as visual perception, speech recognition and decision-making. A more restricted definition, which is germane here, concerns the mimicry of cognitive functions, such as problem solving and learning, also known as machine learning. Machine learning can take the form of training by experts or self-learning by exposure to training data (or both), but involves the ability to self programme in a way that leads to insights or improved performance. And it is this single characteristic that lies at the heart of both the power and the risk of harnessing AI.
THE POWER AND RISK OF AI
AI is already here – search engines, interpreting medical scans, prototype self-driving cars, detection of fraudulent financial transactions, mass surveillance by governments, predicting consumer behaviour, recommending buying choices, and personifying video game characters are just some examples. Likely candidates for AI lie where there are large amounts of input data, some of which may be incomplete or uncertain, and where expert analysis and decision-making is required. Future applications may include air traffic control, driverless transport systems, energy generation and distribution, global manufacturing and supply chains, and the co-ordination of military engagements. Current examples in the leisure and consumer industry are harmless, at worst leading to a poor experience by the end user. But the increasing trial of AI in the healthcare and transport sectors, raises the potential for inadvertent illness, injury or fatality if inappropriately conceived or implemented.
BLACK BOX VS WHITE BOX
In some cases, it may be possible to completely eliminate hazards – for instance, by limiting the motive force of a collaborative robot (or ‘cobot’) involved in a joint manufacturing task. Where this is not practicable, the key to demonstrating AI safety is the emerging concept of ‘explainability’ (Ref. 1), which refers to the idea that AI decision-making should be transparent and explainable – the ‘white box’ approach.
To understand this issue needs an appreciation of the AI’s development process. Like a human, the AI learns through experience and feedback; and like a human is influenced by both its training environment and its trainers. In practice, this means that any training limitations and biases may have unexpected consequences in the real world. In 2018 for instance, it was discovered that cancer treatment advice generated by IBM’s supercomputer Watson was flawed – the cause was attributed to the hypothetical patient data used for training (Ref. 2). Or, when AI was used as an expert system to advise US judges on sentencing, it unwittingly picked up the historical biases of previous offenders, such as ethnicity and gender (Ref. 3). If biases are known, then training data can potentially be cleansed, but in black box systems, any hidden biases will remain hidden.
EXPLAINABLE AI
These issues can be addressed using the white box approach, where the AI logs the underlying factors involved in each decision. In principle, not only does this mean that during training and real world testing any bias or error in judgement can be corrected, it also means that investigation of real world incidents can firmly establish the culpability of the AI and ongoing improvements can be made.
Of course, designing an AI to be explainable has its own problems. A recent application of AI at Moorfield’s Eye Hospital in London speeds up the diagnosis of eye conditions using retinal scans. Cleverly, the system visually identifies the abnormalities used to arrive at its diagnosis, which achieves an accuracy as high as any expert (Ref. 4). What is less clear is how such a system explains a nil result, which is equally important if wrong.
In collaborative safety-critical systems, where a human operator acts as a back-up to an AI control system (or vice versa), as might be employed for train driving for example, it may appear attractive for AI systems to lay out each proposed decision and its reasoning beforehand. For mitigating slowly developing fault conditions, such as reducing maximum speed following the detection of abnormal brake wear, this may be appropriate. For fast-acting situations (such as obstructions on the line), where a rapid response is crucial, there is a clear case for the AI to act without approval. Mixing these two approaches provides another route for erroneous AI decision making.
AI SAFETY ASSURANCE
The fundamental building blocks for demonstrating and maintaining the safe operation of AI systems are well known, at least in outline, since they apply to any software control or protection system:
- Identify and understand the hazards – standard or adapted techniques can be applied to identify the potential impact of component failures (e.g. sensors) and erroneous AI decision making, taking into account training limitations and biases.
- Design ways to eliminate or mitigate hazards, either intrinsically (e.g. by limiting responses physically, or employing redundancy and diversity) or by invoking other systems/operators as defence in depth.
- Design/certify to relevant standards – there are currently no established standards in place for AI safety. The closest available standards are arguably those for functional safety, notably IEC 61508 and ISO 26262, but have significant gaps when applied to AI.
- Demonstrate robustly, but proportionately, that defined safety functions can be met by the AI design, e.g. via its logical modelling architecture, machine learning regime, explainability approach, resilience to faults, processing speed, testing strategy, etc.
- Assess risk both qualitatively and quantitatively against defined safety criteria and consider improvement options – a big issue here is how to predict the reliability of AI decision-making before implementation. One practical way is to rely on the computer simulation of 100,000s of representative scenarios.
- Test as comprehensively as is practicable in a representative (and safe) environment – this may include computer simulation as well as integrated physical testing.
As with any emerging technology the science, techniques, tools and standards ideally needed for AI safety assurance are still developing.
Moreover, with so much progress being made in AI development by so many researchers, it is perhaps too early to expect a consensus on what constitutes best practice. For example, in work undertaken by York University under the auspices of its Assuring Autonomy International Programme, a total of 17 separate approaches to explainability were characterised. Reassuringly, such diversity is probably a sign that developers are serious about building in explainability and serious about building in safety.
CONCLUSION
The future looks set not only to include AI, but to be shaped by it, enabling us to process huge amounts of data and control complex systems quickly and intelligently. Whilst the signs are positive that safety can be baked in from the outset, there remains a good distance to travel before we have all the tools we need to assure AI safety.
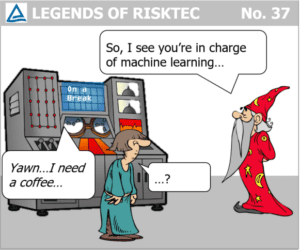
References
- L. H. Gilpin, D. Bau, B. Z. Yuan, A. Bajwa, M. Specter and L. Kagal, “Explaining Explanations: An Overview of Interpretability of Machine Learning,” 2018 IEEE 5th International Conference on Data Science and Advanced Analytics (DSAA), Turin, Italy, 2018, pp. 80-89.
- STAT News, 25 July 2018 (https://www.statnews.com/2018/07/25/ibm-watson-recommended-unsafe-incorrect-treatments).
- AI is sending people to jail—and getting it wrong, MIT Technology Review, 21 Jan 2019.
- De Fauw, J., Ledsam, J.R., Romera-Paredes, B. et al. Clinically applicable deep learning for diagnosis and referral in retinal disease. Nat Med 24, 1342–1350 (2018).