Uncertain Times – Dealing with uncertainty in quantitative risk assessment: A CCS case study
Effective risk-based decision making relies on an accurate characterisation of the likelihood and severity of possible outcomes. This assessment is guided by experience, honed over time as data are gathered and understanding of the risk improves. But what if there is no track record? What if the risk we are assessing is subject to interpretation and based on assumptions? Here we present a real life example of different approaches that have been applied to risk assessment for CO2 leakage from the subsurface storage reservoir of a Carbon Capture and Storage (CCS) project.
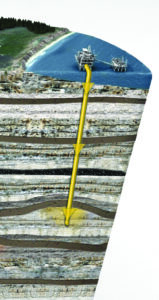
RISK IN RELATION TO RESOURCE
By definition, risk-based decisions are made on the basis of an estimate of the risk. We devote more energy to reducing the highest risks – particularly where they are found to exceed tolerability thresholds (e.g. the red lines in Figure 1) – confident that we are directing our resources efficiently.
Risk ranking is often quantitative, such that the calculated risk can be compared to these threshold values. However, for any quantification, inevitably there are underpinning assumptions or analyses that have uncertainties attached. It is important to address these uncertainties to ensure we have good confidence that the underlying risk is faithfully represented, and that the efforts to manage it are, in fact, proportionate.
THE UNCERTAINTY ISSUE
Uncertainty is always present when anything is evaluated or estimated. This is especially the case for risk in new endeavours, where novel technologies are implemented or new hazardous activities are undertaken.
Take, for example, the risk of leakage of CO2 from a subsurface storage reservoir. With the CO2 storage industry in its infancy, there is only limited operational experience from a handful of storage sites. Coupled with the already tricky task of characterising and predicting the behaviour of geological structures several kilometres below the surface, CO2 leakage risk assessment is riddled with uncertainty.
One way to handle uncertainty is to err on the side of caution and make conservative simplifying assumptions. This builds in a safety factor and provides reassurance, however it tends to inflate the risk level and may invite excessive scrutiny of the risk (e.g. from regulators or stakeholders), when in fact the actual risk is low. At the same time, it can also lead to a disproportionate allocation of effort and cost in attempting to reduce the risk.
UNCERTAINTY IN CO2 CONTAINMENT RISK ASSESSMENT
In the relatively short operational history of CCS there have been several incidents at storage sites. These issues have been predominantly associated with mischaracterisation of the subsurface environment – which is uncertain owing to its heterogeneity and anisotropicity – due mostly to a lack of data. For example:
- At Snøhvit, Norway, injectivity was lower than predicted due to the uncertain nature of the geology. This resulted in a costly redesign with the injection targeting a different section of the reservoir (Ref. 1)
- At In Salah, Algeria, the unrevealed presence of a fault and fracture network in the caprock led to ground uplift surrounding the injection site and the early termination of injection (Ref. 2)
Evidently, unfavourable outcomes can result when:
- The risk is not identified and therefore not managed
- The risk is incorrectly assessed as acceptably low, and therefore not examined in sufficient detail
Quantifying the uncertainty in the estimation of a risk – which is a requirement of ISO 27914 (Ref. 3) – helps us understand our confidence that the risk is not higher (or lower) than expected.
EXPERT JUDGEMENT OF CO2 LEAK RISK
One typical scenario relates to CO2 leaking through a fault, eventually reaching the sea bed through overlying rock. For the scenario to occur the reservoir injection pressure limits must be exceeded, the fault must act as a conduit to CO2 flow, and secondary impermeable layers above the caprock must also fail to contain the CO2.
How can a numerical estimate of risk be derived?
TOP-DOWN SINGLE POINT EXPERT JUDGEMENT
A starting point is to use expert consensus based on the pre-defined categories of a risk matrix. The likelihood and severity both lie within a range, corresponding to one box on the matrix; as indicated by the black dot in Figure 1, i.e. ‘remote’ probability and ‘serious’ consequence.
It is clear that this box is in the ‘low’ risk band, but what about the uncertainty in this decision? One category either way? More? Less? By using order of magnitude categories we are constraining our estimate.
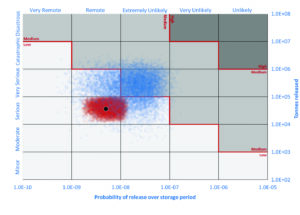
Figure 1 – Expert judgements of CO2 leak risk
TOP-DOWN THREE POINT EXPERT JUDGEMENT
Rather than picking a single value for a parameter, it is perfectly possible for assessors to define their own range with minimum and maximum values. Together with the central best-estimate value, this defines a triangular probability distribution. This emphasises the central value and gives less weighting to the low and high extremes, reflecting the judgements made. The β-PERT distribution uses the same three inputs, but is preferred because it produces a smoother distribution that is a more realistic representation of a real world parameter.
Using Monte Carlo simulation we can sample from the distribution and produce a range of results. For example, applying a most-likely estimate with an order of magnitude range for the minimum and maximum values to both the likelihood and total mass released, 10,000 simulations produces the red cloud of values in Figure 1.
The top-down three-point expert judgement predicts a 91% chance that that the risk is ‘serious’ and ‘remote’ (as per the initial single point judgement), but note how some of the simulations result in an ‘extremely unlikely’ probability, and/or a ‘very serious’ consequence. Overall, 99% of the simulations predict a low risk.
BOTTOM-UP MODELLING
For a leak path to exist or develop, several barriers must fail. We can apply an approach akin to Layer of Protection Analysis (LOPA) or event tree analysis by multiplying the likelihood of the leak occurring by the probabilities with which the barriers are estimated to fail.
Similarly, the estimated release rate (tonnes per year) and duration (years) can be estimated and multiplied together to yield the total mass lost.
We can extend the idea of parameterising expert judgement through the β-PERT distribution to each of these parameters, resulting in a bottom-up quantification of the risk.
The results of 10,000 simulations are seen as the large, blue cloud of values in Figure 1. The simulations are even more spread out, reflecting the compounding of the uncertainties by multiplying the tails of several parameter distributions. Just over half of the simulations have crept into the medium risk zone, and about 50% are in the ‘very serious’ category. We have to conclude that our original single point expert judgement, and even the three point approach, underestimated the true risk.
CONCLUSION
Where data are scarce and expert judgement is required to interpret risks, we should account for the uncertainty explicitly in our estimation of risk.
Taking CCS as a case study for trialling top-down and bottom-up estimations of CO2 leakage risk, it becomes clear that as uncertainty analysis becomes more refined it can have a significant impact on conclusions.
This article first appeared in RISKworld 41, issued April 2022.
References:
- Shi et al., Snøhvit CO2 Storage Project: Assessment of CO2 Injection Performance through History Matching of the Injection Well Pressure Over a 32-months Period, 2013
- Ringrose et al., The In Salah CO2 Storage Project: Lessons Learned and Knowledge Transfer, 2013
- ISO 27914:2017, Carbon dioxide capture, transportation and geological storage – Geological storage